Latex Equations From Model Objects the Equatiomatic Package
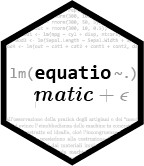
As I was building a recent preprint, and trying to translate a long Bayesian formula (courtesy the big brain of Scott Mourtgos) into properly specified LaTeX, I thought there has to be a better way. As usual, my decision to follow Andrew Heiss’ github paid off, as I saw he has been authoring the equatiomatic
package. The project is maintained by Daniel Anderson, and you can check it out yourself here.
The beauty of equatiomatic
is clear - it takes your model object in R and translates it into beautifully rendered LaTeX equations.
Thought I’d quickly demo the package using some easy data I had laying around.
Walking Through equatiomatic
First get the package installed and loaded:
# package install
# install.packages("equatiomatic", repos = "http://cran.us.r-project.org")
# load up
library(equatiomatic)
I’m using some data from my most recent publication in Public Administration Review, which tests competing theories of body-worn camera (BWC) activation. We ask: Is variation in BWC activations more explained by officer attitudes towards the cameras, by officer demographics, or by job function. I won’t repeat the whole analysis here, but you can find out by visiting the article!
library(tidyverse)
activations <- read_csv("activations.csv")
head(activations)
## # A tibble: 6 x 26
## totalactivations Activ_Plus_One_~ years_LEO Female rank BWC_time forcecount
## <dbl> <dbl> <dbl> <dbl> <dbl> <dbl> <dbl>
## 1 54 4.01 4 0 1 5 0
## 2 79 4.38 10 0 1 3 0
## 3 138 4.93 4 0 1 5 1
## 4 11 2.48 11 0 1 5 0
## 5 148 5.00 20 0 1 5 0
## 6 198 5.29 3 0 1 4 1
## # ... with 19 more variables: totalprimarycalls <dbl>, arrests <dbl>,
## # Line_Officer <dbl>, BWCapproval_new <dbl>, POS_LATENT <dbl>,
## # BWC_understand <dbl>, BWC_freedom <dbl>, BWC_decision <dbl>,
## # BWC_manipulate <dbl>, BWC_modify <dbl>, BWC_lessforce <dbl>,
## # BWC_assault <dbl>, BWC_complaint <dbl>, BWC_personal <dbl>,
## # BWC_embarrass <dbl>, BWC_hatred <dbl>, BWC_fair <dbl>, BWC_protect <dbl>,
## # BWC_wellbeing <dbl>
Let’s build a quick (and misspecified here) version of one of the main models of interest in the paper:
job_function <- lm(totalactivations ~ forcecount + totalprimarycalls + arrests + Line_Officer, activations)
Now, we give the results of that model to equatiomatic
and let it extract and build:
equatiomatic::extract_eq(job_function,
wrap = TRUE, # Long equation needs to wrap
terms_per_line = 2) # Max two equation terms per line
## $$
## \begin{aligned}
## \operatorname{totalactivations} &= \alpha + \beta_{1}(\operatorname{forcecount})\ + \\
## &\quad \beta_{2}(\operatorname{totalprimarycalls}) + \beta_{3}(\operatorname{arrests})\ + \\
## &\quad \beta_{4}(\operatorname{Line\_Officer}) + \epsilon
## \end{aligned}
## $$
We can take the output directly to Rmarkdown using the given LaTeX!
\[ \begin{aligned} \operatorname{totalactivations} &= \alpha + \beta_{1}(\operatorname{forcecount})\ + \\ &\quad \beta_{2}(\operatorname{totalprimarycalls}) + \beta_{3}(\operatorname{arrests})\ + \\ &\quad \beta_{4}(\operatorname{Line\_Officer}) + \epsilon \end{aligned} \]
Absolutely gorgeous! But it gets better, we can include the coefficients instead of funny Greek letters!
equatiomatic::extract_eq(job_function,
use_coefs = TRUE, # Use coefficients instead of beta
wrap = TRUE, # Long equation needs to wrap
terms_per_line = 2) # Max two equation terms per line
## $$
## \begin{aligned}
## \operatorname{totalactivations} &= 6.19 + 10.66(\operatorname{forcecount})\ + \\
## &\quad 0.65(\operatorname{totalprimarycalls}) + 3.91(\operatorname{arrests})\ + \\
## &\quad 18.78(\operatorname{Line\_Officer}) + \epsilon
## \end{aligned}
## $$
Again, copy/paste over the LaTeX given by equatiomatic, and:
\[ \begin{aligned} \operatorname{totalactivations} &= 6.19 + 10.66(\operatorname{forcecount})\ + \\ &\quad 0.65(\operatorname{totalprimarycalls}) + 3.91(\operatorname{arrests})\ + \\ &\quad 18.78(\operatorname{Line\_Officer}) + \epsilon \end{aligned} \]
By the way, the package isn’t limited to linear regressions, and already has support for logistic and probit regressions with glm()
, and ordered logistic regressions. Hit up the package home to follow development.
I am completely impressed by this young package so far, and can’t wait to see what else is coming!